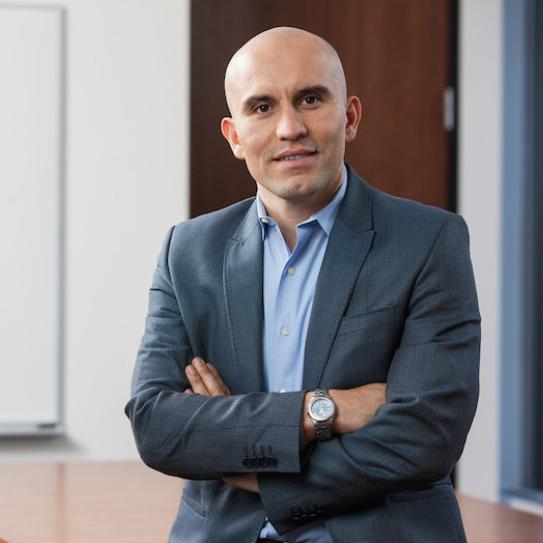
JeanCarlo (J.C.) Bonilla has over 20 years of blended experience in data science for higher education. At NYU, he has managed multiple recruitment, admissions, and academic services initiatives. Currently, J.C. serves as the Chief Analytics Office at Element451, where he leads the "intelligent admissions" practice across 60+ universities. This practice consists of the deployment of machine learning and predictive modeling technology for enrollment management, building advanced analytics use cases and supporting insight generation capabilities for all schools. In addition, J.C. is a faculty member at NYU, where he teaches Business Analytics and Analytics for Process Improvement
J.C. Bonilla holds and Bachelor's and Master's degrees in Electrical Engineering and a Ph.D. in Technology Management from New York University.
Education
- Ph.D., Technology Management, New York University, NY
- M.S. Electrical Engineering, New York University, NY
- B.S. Electrical Engineering, John Brown University, AR
Courses
Business Analytics
Business analytics is a set of data analysis and modeling techniques for understanding business situations and improving business decisions. This course provides an introduction to business analytics concepts, methods and tools with concrete examples from industry applications. In the first part of the course, we will focus on descriptive analytics and exploratory data analysis concepts with a refresher on basic probability and statistics. In the second part, we will cover principles, techniques, and techniques for spatial data, time series, and text as data. The final part of the course will introduce a project that links business impact and modern data analytics techniques for managerial decision making in functional areas, including finance, marketing, and operations.
Throughout the course, we explore the challenges that can arise in implementing analytical approaches within an organization. The course emphasizes that business analytics is not a theoretical discipline: these techniques are only interesting and important to the extent that they can be used to provide real insights and improve the speed, reliability, and quality of decisions.
Data Analytics for Process Improvement
Analytics for process performance is a more specific term for a type of business analytics which focuses on improving existing operations. This type of analytics involves the use of various data mining and data aggregation tools to get more transparent information for business planning, develop consensus and drive improvements.
This course provides an introduction to process analytics concepts, methods and tools with concrete examples from industry applications. This course builds around 3 unique projects: First, a project on descriptive analytics to measure and analyze processes. In this first part of the course, we will focus on descriptive analytics and exploratory data analysis concepts with a refresher on basic probability and statistics. The second project involves predictive analytics for process performance. Here, we will cover predictive techniques such a linear regression, classification modeling, and text mining. The final project is the capstone experience of the course. Using publicly accessible datasets or data that is secured via mutual consent, students are required to link business impact and modern data analytics techniques for overall process improvement.
Throughout the course, we explore the challenges that can arise in implementing analytical approaches within an organization. The course emphasizes that analytics is not a theoretical discipline: these techniques are only interesting and important to the extent that they can be used to provide real insights and improve the speed, reliability, and quality of operations.