Closed-loop tracking and regulation of emotional valence state from facial electromyogram measurements
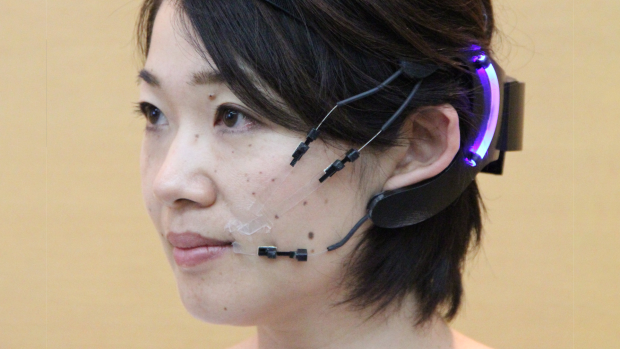
Emotions directly influence the way we think and interact with others in different situations. Additionally, when it interferes with rationality in our decision-making or perception, emotions play an undeniable role.
From another perspective, studies from different fields indicate that human emotions could be impacted by any changes in the environment. Thus, having a solid understanding of the dynamics of emotions could benefit individuals in multiple ways: from the enhancement of therapeutic solutions and maintaining well-being to the prevention of unpleasant emotions and inducing favorable ones. Decoding emotions has been an ongoing research among researchers, dictating joint efforts from behavioral, physiological, and computational angles.
According to Russell's circumplex model, emotions are defined in a two-dimensional arousal-valence circular space. Compared to the arousal index, which designates the severity of the emotional state, internal valence levels determine the quality of emotions. In a high arousal mood, feeling excited or happy is opposed to nervousness and feeling stressed. On the flip side and in low arousal conditions, staying calm stands on the opposite side of feeling bored. Hence, feeling pleasant, which refers to the positive valence, is one of the key factors in one’s health condition and could result in a better quality of life.
In a new piece of research, a team led by Rose Faghih, Associate Professor of Biomedical Engineering, follows the goal of establishing a mechanism to track an individual's emotional valence level and regulate it in a closed-loop framework. Since the emotional valence state is hidden and cannot be measured directly, the authors need to measure it indirectly.
The use of wearable devices and designing decoders to gain insight into the internal brain states provides a good alternative to directly measuring brain activity. Inspired by recent advances in wearable technologies which could measure various physiological signals from humans in the loop, there exists possibilities to track emotional valence state indirectly and in a more practical manner. Among different physiological signals, it has been shown that facial electromyography (EMG) carries valuable information about the internal valence state. According to various research, facial EMG measurements of the zygomaticus muscle (zEMG) give the most distinct indicator of emotional valence state. In fact, changes in the zygomaticus muscle, which could be captured via wearables, could provide researchers with valuable information regarding one’s valence level.
In this research, the authors employ a mathematical framework to relate changes in zEMG signal for inferring the internal valence state. They extract corresponding features from the zEMG signal and estimate the internal valence state. Taking advantage of tracking of emotional valence state in a systematic way, they then propose a knowledge-based control architecture to close the loop and keep the estimated valence state within a favorable range. Within a closed-loop approach, biomarkers are collected to provide information regarding the individual's valence state to then automatically adjust the actuators in response to the current emotional state.
In silico results verify that, by making use of physiological data, the proposed controller could effectively regulate the estimated valence state. Ultimately, it is envisioned that the future outcomes of this research will include supporting alternative forms of self-therapy by using wearable machine interface architectures capable of mitigating periods of pervasive emotions and maintaining daily well-being and welfare. This study is the very first closed-loop control framework for regulating emotional valence state using facial muscle activity. This research suggests an important new step toward new clinical applications and the self-management of mental health. In the practical implementation of the proposed architecture, a wearable device would collect zEMG signal, and the appropriate practices (e.g., listening to music, breathing exercises) would be suggested to maintain the emotional valence levels within the favorable range.
Faghih, who joined NYU earlier in 2022, has been integrating research into her classroom teaching. The research covered in this Frontiers in Computational Neuroscience paper started as a course project in Faghih’s “State- Space Estimation with Physiological Applications” course at the University of Houston. In this class students had the opportunity to use advanced methods learned in the course to analyze physiological data in hands-on biomedical engineering projects designed by Faghih. Student authors Luciano Branco and Arian Ehteshami were graduate students in the course, and Hamid Fekri Azgomi, who recently completed his PhD under the supervision of Faghih, was a PhD student mentor for the project.