Visualizing Better Healthcare
Tandon Engineers Are Partnering With Clinicians to Help Make Disease Prediction, Diagnosis, and Treatment More Effective Than Ever

Professors in Tandon’s Department of Biomedical Engineering are carrying on a long, global tradition of innovation in medical imaging. Since 1895 — when German physicist Wilhelm Röntgen made the startling discovery that he could obtain an image of the bones in his wife’s hand by placing it over a photographic plate in the path of cathode rays — we have generally come to take X-rays for granted. With computed tomography (CT) scans providing even more details, it’s tempting to believe that the human body has few mysteries left to unveil.
But as clinicians strive to better diagnose conditions, predict disease progression, and formulate treatment plans, there are still many medical mysteries yet to be solved. Advances in imaging techniques and improvements in how those images can be visualized and interpreted are proving to be key, and Tandon is making enormous contributions in that arena.
Joel S. Schuman
Ophthalmologist Joel Schuman wears many hats. He serves as the director of NYU Langone’s Eye Center and chair of Langone’s Department of Ophthalmology and holds a professorship, as well, in the Department of Neuroscience and Physiology — all in addition to his work as a core faculty member in Tandon’s Department of Biomedical Engineering.

Schuman’s research interests include the imaging of the eye and visual system, regenerative ophthalmology, aqueous outflow, and laser-tissue interactions, and he achieved renown as a creator of optical coherence tomography (OCT), a non-invasive, high-resolution in vivo imaging device for the diagnosis and management of eye disease. OCT is now considered the standard of care worldwide, making Schuman a revered figure in the world of ophthalmology. And if that’s not enough, he and his team were also the first researchers ever to identify a molecular marker for human glaucoma. Such landmark accomplishments would not have been possible, he says, without the input of engineers, and he works closely with those from a variety of disciplines, including Ivan Selesnick, chair of Tandon’s Department of Electrical and Computer Engineering (see below), and Guido Gerig, Chair and Institute Professor in the Department of Computer Science and Engineering, as well as a member of Tandon’s Visualization and Data Analytics (ViDA) Laboratory.
Among Schuman’s latest projects is the use of visible light OCT (vis-OCT) — a tool that allows for improved imaging resolution — and he’s also exploring the relevance of deep-learning techniques to medical imaging and new methods of applying 3D processing techniques to OCT data. “Working together, engineers and medical researchers can develop disruptive technology for the early detection of disease and find new ways to predict its progression,” he says. “Our common goals are improved healthcare and better patient outcomes.”
Ivan Selesnick
Department of Electrical and Computer Engineering Chair Ivan Selesnick is an expert in digital signal processing, among other areas, and he and his team have developed new signal processing algorithms for numerous types of data and applications — including such biomedical imaging methods as near infrared spectroscopy (NIRS), OCT (see above), magnetic resonance imaging, digital x-ray imaging, electrocardiography, and electroencephalography.
“Working with medical researchers, we learn about problems in data analysis that we might never have known about or considered before, and we’re finding ways to apply our algorithms to projects of great potential benefit to patients.”
One of those projects, undertaken in collaboration with department colleague John-Ross Rizzo and other NYU Langone researchers, has involved the development of a new algorithm that boosts the accuracy of the King–Devick (KD) test, an assessment based on eye movement that is frequently used to screen sidelined athletes for possible concussion. Selesnick is quick to credit Weiwei Dai, one of his doctoral students, for making important contributions to the research, and speaking to him for any length of time, it becomes apparent: Selesnick shines in his role as mentor and advisor and takes great pride in the many accomplishments of his students — some working on issues in medical imaging and some tackling other health-related matters.
Among those also doing praise-worthy work is Roozbeh Soleymani, who has created an innovative noise reduction technology called SEDA (Speech Enhancement using Decomposition Approach), in collaboration with Selesnick (whose earlier National Science Foundation-funded research wahttps://engineering.nyu.edu/student/roozbeh-soleymanis the springboard for Soleymani’s work) and David Landsberger of Langone’s Department of Otolaryngology. SEDA has huge potential to improve the lives of those who use cochlear implants and hearing aids and who often have difficulty teasing out what someone is saying over “babble” — the cacophony of other talkers — and other ambient sounds. “An electrical engineer developing this type of algorithm might initially think of its application to cell phone users or in similar market-driven sectors,” Selesnick says, “but by working with medical researchers, Roozbeh has been able to contribute to the social good in a different and meaningful way.”
Daniel Sodickson
Daniel Sodickson — whose titles include professor of radiology, professor of neuroscience and physiology, and vice chair for research in the Department of Radiology at NYU Langone, as well as director of the Bernard and Irene Schwartz Center for Biomedical Imaging, in addition to his post in Tandon’s Department of Biomedical Engineering — is a seminal figure in the development of parallel magnetic resonance imaging (MRI), which involves the use of radiofrequency (RF) coil arrays to acquire data in parallel rather than in a traditional sequential fashion and which enabled imaging at previously inaccessible speeds.

Countless patients are likely to be unaware that they owe him a debt of gratitude for that development. Placed in a noisy scanner for as long as an hour (depending on the part of the body being imaged), many complain about the discomfort and sense of confinement that often accompany the test. Sodickson acknowledges as much; during a recent lecture he played an audio recording of the discomfiting sounds to which MRI patients are subjected in order to drive home the importance and benefit of his latest research: using machine learning techniques to make MRI scans even quicker and thus less uncomfortable.
Sodickson, who holds a Ph.D. in medical physics in addition to his M.D., has overseen NYU’s development of a multifaceted translational imaging research program and the founding of its Center for Advanced Imaging Innovation and Research, which is aimed at developing novel imaging technologies for the improved management of cancer, musculoskeletal disease, and neurological disease — and at getting those innovations quickly from lab bench to patient bedside. His overarching goal, he explains, “is to change the paradigms of data acquisition, image reconstruction, and day-to-day scanning in biomedical imaging, for the benefit of patients and the physicians who care for them.”
Yao Wang
Yao Wang, a professor of electrical and computer engineering as well as biomedical engineering, is using machine learning to deliver strong medicine to health care research, diagnostics, self-therapy, and ultimately, prognosis.
Along with Mei Fu, an associate professor at NYU Rory Meyers College of Nursing, she has devised a way to detect and manage lymphedema, an incurable obstruction or disruption of the lymphatic system that is characterized by swelling of one or more limbs and that is common after breast cancer surgery. They have developed a training system for patients performing lymphatic exercises designed by Dr. Fu, which have been shown through clinical trials to improve the lymph flow and reduce the risk for progressing to chronic lymphedema. The system uses a Kinect sensor to follow the patient's movement and provides instant feedback to the patients, to improve the efficacy of the exercises.
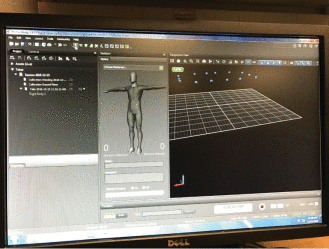
Software interface of the motion capture system used to develop an exercise system for lymphedema intervention
The Optimal Lymph-Flow (TOLF) system is far from the only medical collaboration in which Wang is involved. She is also investigating the uses of machine learning for the detection and outcome prediction of mild traumatic brain injury (MTBI) using advanced MRI imaging features in collaboration with Dr. Yvonne Lui at the Department of Radiology; leveraging ultra-high frequency ultrasound images of mouse embryos to analyze defects caused by genetic mutations in collaboration with Dr. Daniel Turnbull from the Departments of Radiology and Pathology and colleagues at Riverside Research Institute; and examining intracranial electrophysiological recording to gain a better understanding of which cortical region is responsible for functions like audio perception, semantic understanding, speech planning, and speech production with Dr. Adeen Flinker from the Department of Neurology; among other such projects.
Working at a large university like NYU, with a world-class medical school, presents ample opportunity for collaboration and for creating technology that can be used to serve humanity,” she says. “It’s exciting to be doing work that has immediate benefits for patients and the potential to further medical understanding in decades to come.”