John-Ross Rizzo
,
MD
-
Rehabilitation Medicine, Vice Chair for Innovation & Equity, Ilse Melamid Endowed A/Prof of Rehab Medicine, Associate Professor of Neurology, Mechanical & Aerospace Engineering, and Biomedical Engineering
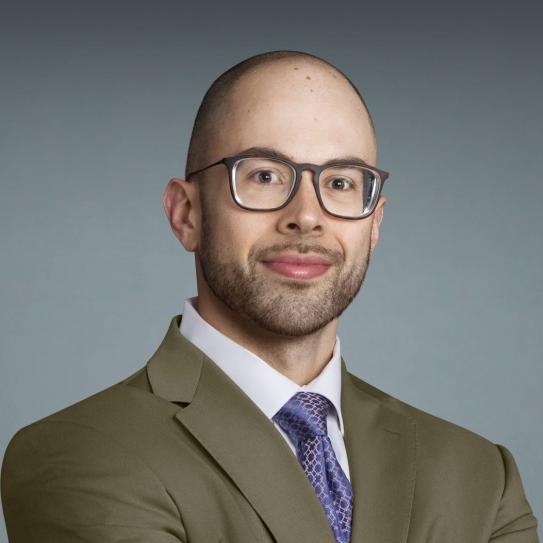
- Director, Visuomotor Integration Laboratory
- Director, Rehabilitation Engineering Alliance and Center Transforming Low Vision
Advances in miniaturized sensors and actuators, as well as artificial intelligence (AI), have broadened horizons for assistive and rehabilitative technologies. The team of Professor JohnRoss Rizzo, MD, is leveraging these innovations to help patients with conditions such as blindness and stroke, enhancing their ability to interact physically with their environment.
His research is supported in part by NYU WIRELESS.
Education
New York Medical College
MD
NYU Langone Medical Center, Rusk Rehabilitation, Clinical Research Fellowship
Fellowship
NYU Langone Medical Center, Rusk Rehabilitation (with Chief Year)
Residency
Experience
New York University
Assistant Professor, Department of Rehabilitation Medicine
Assistant Professor, Department of Neurology
Director, Visuomotor Integration Laboratory
Research News
New app developed at NYU Tandon promises to make navigating subway stations easier for people with blindness and low vision
A new trip-planning app has shown encouraging results in improving navigation inside subway stations, according to a study published in IEEE Journal of Translational Engineering in Health and Medicine, promising the possibility of easier commutes for people who are blind and low-vision.
Designed by researchers at NYU Tandon School of Engineering and NYU Grossman School of Medicine, Commute Booster routes public-transportation users through the “middle mile” — the part of a journey inside subway stations or other similar transit hubs — in addition to the “first” and “last” miles that bring travelers to and from those hubs.
“The ‘middle mile’ often involves negotiating a complex network of underground corridors, ticket booths and subway platforms. It can be treacherous for people who cannot rely on sight,” said John-Ross Rizzo, MD, who led the research team that includes advisors from New York City’s Metropolitan Transit Authority (MTA). Rizzo is an associate professor in NYU Tandon’s Biomedical Engineering department and is on the faculty of NYU Grossman. “Most GPS-enabled navigation apps address ‘first’ and ‘last’ miles only, so they fall short of meeting the needs of blind or low-vision commuters. Commute Booster is meant to fill that gap.”
Subway signs are typically graphical or text-based, creating challenges for the visually impaired to recognize from distances and reducing their ability to be autonomous in unfamiliar environments.
Commute Booster automatically figures out what signs a traveler will encounter along the way to a specific subway platform. Then, it uses a smartphone’s camera to recognize and interpret signs posted inside transit hubs, ignoring irrelevant ones and prompting users to follow relevant ones only.
In the recent study, researchers tested Commute Booster’s interpretation of signage from three New York City subway stations — Jay Street-Metrotech, Dekalb Avenue and Canal Street — that a traveler would encounter on a specific journey. The app proved 97 percent accurate in identifying signs relevant to reach the intended destination.
Testing inside those three subway stations also revealed that Commute Booster could “read” signs from distances and at angles that reflect expected physical positioning of travelers.
The Commute Booster system relies on two technological components. The first, general transit feed specification (GTFS), is a standardized way for public transportation agencies to share their transit data with developers and third-party applications. The second, optical character recognition (OCR), is technology that can translate images of text into actual editable text.
The GTFS dataset contains descriptions for locations and pathways within each subway station. Commute Booster’s algorithm uses this information to generate a comprehensive list of wayfinding signage within subway stations that users would encounter during their intended journey. The OCR functionality reads all texts presented to users in their immediate surroundings. Commute Booster’s algorithm can identify relevant navigation signs and locate the position of signs in the immediate environments. By integrating these two components, Commute Booster provides real-time feedback to users regarding the presence or absence of relevant navigation signs within the field of view of their phone camera during their journey.
Researchers plan to conduct a human subject study of Commute Booster in the near future. The app could be available for public use in the near term.
Rizzo, who was named to MTA’s board in June 2023, has a long track record of research that applies engineering solutions to challenges faced by people with disabilities, particularly those with visual disability .
In addition to Rizzo, the team involved in the Commute Booster study are NYU Tandon PhD candidate Junchi Feng; Physician-Scientist at NYU Langone’s Rusk Rehabilitation Mahya Beheshti; MTA Senior Innovation Strategist Mira Philipson; MTA Senior Accessibility Officer Yuvraj Ramsaywack; and NYU Tandon Institute Professor Maurizio Porfiri.
This research was supported by the National Science Foundation, the National Eye Institute and Fogarty International Center, as well as by the U.S. Department of Defense.
Fostering innovation by connecting engineering and medical students
A new paper from researchers at NYU Tandon School of Engineering and the NYU Grossman School of Medicine explores how interdisciplinary programs connecting medical and engineering education may foster innovation and prepare students in both disciplines for more successful careers.
The paper, published in the Technology and Innovation journal of the National Academy of Inventors, describes initiatives at NYU as a case study, along with similar programs at Johns Hopkins University, Stanford University, Harvard and Massachusetts Institute of Technology.
NYU Tandon and Grossman have partnered on educational programs for about a decade. But for most of this time, the skill-sharing only went in one direction, explained lead author John-Ross Rizzo, a rehabilitation medicine specialist and professor at both schools. “It dawned on us that we spend a ton of time bringing engineers to the medical school, but almost zero time trying to get our doctors immersed in the engineering world,” Rizzo said.
By bringing medical students to the engineering field, as NYU has done in recent years, educators can enable a shared understanding of engineering concepts that contributes to more effective problem-solving, Rizzo and his colleagues argue. Clinicians and engineers are more capable of collaboration if they speak each other’s languages; new innovations that result from these partnerships may be better set up for long-term success.
Rizzo compared this interdisciplinary learning to earning belts in martial arts. A medical student might not become a “black belt in computer science,” but might learn enough for a “yellow belt” — a lower level of understanding, but enough to enable collaboration with the true experts. “We’re creating a smarter generation of students,” Rizzo said.
One way NYU students may gain this expertise is through participation in the NYU HealthTech Transformer Challenge, which pairs engineers and clinicians to work on “healthcare’s most pressing problems.” Finalists from the program have won funding from NYU and other sources to pursue their ideas at new startups. Other challenges and grant-funded research projects at NYU Tandon and Grossman have allowed graduate students to receive co-advising from engineering and medical professors.
The researchers also discussed barriers to setting up these interdisciplinary programs. Early initiatives may require extensive effort, including dedicated advocacy to bring different school administrators on board. It may be especially tough to convince medical school leaders to devote student time to engineering work outside their typical course load. Part of the challenge is a lack of data: while NYU and similar programs have produced some clear success stories among individual students and startups, universities are not tracking their results in a comprehensive manner.
In the new paper, Rizzo and colleagues share lessons from NYU’s leadership in this interdisciplinary space and from programs at other institutions. The findings may provide inspiration for more universities to consider connecting medicine and engineering education. “I think this is a trend we’ll hear more about over the next decade,” Rizzo said.
Brain-Muscle Connectivity Network for Assessing Stroke NeuroRehabilitation
This project is led by S. Farokh Atashzar, assistant professor of electrical and computer engineering at NYU Tandon; and John-Ross Rizzo, assistant professor in the Departments of Rehabilitation Medicine and Neurology at NYU Langone Health, and of mechanical and aerospace and biomedical engineering at NYU Tandon.
Stroke, the leading cause of motor disabilities, is putting tremendous pressure on healthcare infrastructures because of an imbalance between an aging society and available neurorehabilitation resources. Thus, there has been a surge in the production of novel rehabilitative technologies for accelerating recovery. Despite the successful development of such devices, lack of objective standards besides clinical investigations using subjective measures have led to controversial recommendations regarding several devices, including robots.
This NSF/FDA Scholar-in-Residence project, designed to address the need for effective rehabilitative technologies, is focused on the design, implementation, and evaluation of a novel, objective, and robust algorithmic biomarker of recovery. Called Delta CorticoMuscular Information-based Connectivity (D-CMiC), the proposed algorithm-based protocol quantifies the connectivity between the central nervous system (CNS) and the peripheral nervous system (PNS) by simultaneously measuring electrical activity from the brain and an ankle muscle on the affected side of recovering post-stroke patients. The system will quantify both spectrotemporal neurophysiological connectivity between the CNS (using electroencephalography (EEG) and PNS (using high-density surface electromyography (HD-sEMG).
The goal of the collaborative project, beyond clarifying the neurophysiology of recovery, is to expedite availability of more effective rehabilitation devices to patients for a range of neurological disorders beyond stroke (such as Parkinson's disease, Essential Tremor and Ataxia). For educational impact, the project will generate a unique transdisciplinary educational environment by conducting workshops about emerging Brain-Computer Interface (BCI) technologies in medicine, and undergraduate team projects for human-machine interfacing, with a focus on promoting STEM activities within underrepresented groups.
The predictive capability, precision, and efficiency of the developed D-CMiC metric will be analyzed by collecting data from recovering stroke patients and healthy subjects alike. Unique D-CMiC features include: (1) accurately and objectively tracking corticomuscular functional connectivity in the Delta/low frequency band; (2) computationally modeling of corticomuscular connectivity; (3) building the basis for the first medical device development tool for the systematic, objective, and transparent evaluation of pre-market rehabilitation devices, aligned with the FDA's mission.